Invited Speaker
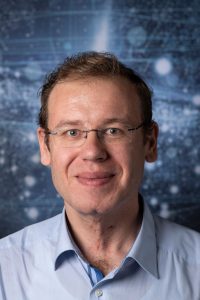
Prof. Dr. Andreas Maier
FAU Erlangen-Nürnberg, Department Digital Humanities and Social Studies
Topic: Known Operator Learning and Hybrid Machine Learning in Medical Imaging - The Past, the Present, and the Future
Abstract: In this presentation, we perform a review of the state-of-the-art of hybrid machine learning in medical imaging. We start with a short summary of the general developments of the past in machine learning and how general and specialized approaches have been in competition in the past decades. A particular focus will be the theoretical and experimental evidence pro and contra hybrid modelling. Next, we inspect several new developments regarding hybrid machine learning with a particular focus on so-called known operator learning and how hybrid approaches gain more and more momentum across essentially all applications in medical imaging and medical image analysis. As we will point out by numerous examples, hybrid models are taking over in image reconstruction and analysis. Even domains such as physical simulation and scanner and acquisition design are being addressed using machine learning grey box modelling approaches. Towards the end, we will investigate a few future directions and point out relevant areas in which hybrid modelling, meta learning, and other domains will likely be able to drive the state-of-the-art ahead.
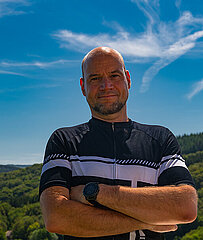
Prof. Dr. Ingo Scholtes
University of Wuerzburg, Machine Learning for Complex Networks
Topic: How the Arrow of Time shapes the Causal Topology of Temporal Graphs - From Modelling to Deep Learning
Abstract: Graph Neural Networks have become an important paradigm in the application of machine learning to data on complex systems with many interacting elements. Apart from relational data that captures which of a system's elements are connected, we increasingly have access to high-resolution time series data that captures when and in which order those connections occur. Due to the arrow of time, the temporal order of those connections shapes the causal topology of temporal graphs, i.e. which nodes can possibly causally influence each other over time. This leads to non-trivial effects that must be accounted for in the modelling of dynamical processes in graph with dynamic topologies. Addressing this issue, I will show that the spectral properties of higher-order De Bruijn Graphs can help us to better understand how the arrow of time influences the evolution of dynamical processes in temporal graphs. Apart from improving our understanding of social, technical and biological networks with dynamic topologies, these models are the theoretical foundation for De Bruijn Graph Neural Networks, a new time-aware deep learning architecture for temporal graph data. Accounting for temporal-topological patterns in temporal graphs, our approach facilitates deep graph learning in time series data on complex networks.